

ILP: How Sample Size and Distribution of Parent Population Affect the Sampling Distribution of the Sample Mean Which graph below best represents the model that could be used to approximate the probability that the average waiting time is at most 5 minutes? Suppose a random sample of 100 drive-‐through window orders will be selected from over the past week and the average waiting time for placing the orders will be calculated. The mean waiting time is 4 minutes and the standard deviation is 2.3 minutes. Suppose the time to wait for placing an order at a drive-‐through window has a uniform distribution between 0 and 8 minutes. We will next examine the sampling distribution of the sample statistic most commonly used for measuring the center of a distribution -‐-‐ the sample mean. Thus, we would prefer Estimator I to Estimator II. Generally, if we use a statistic to make an inference about a population parameter, we want its sampling distribution to be centered at the true parameter (a characteristic which allows us to call that statistic unbiased), and we would like variability in the estimates to be as small as possible.īelow, we have two estimators that are both unbiased, but Estimator I has less variability (is more precise). The probability distribution of the sample statistic is called its sampling distribution. When data are gathered by random sampling, the statistic will be a random variable and as such, it will have a probability distribution. This can be seen more easily through some pictures: With a large number of samples, you can assess whether the value of the statistic (e.g., sample mean, X ) will frequently be close to the true value of the population parameter (e.g., population mean, μ), and if so, how close on average.
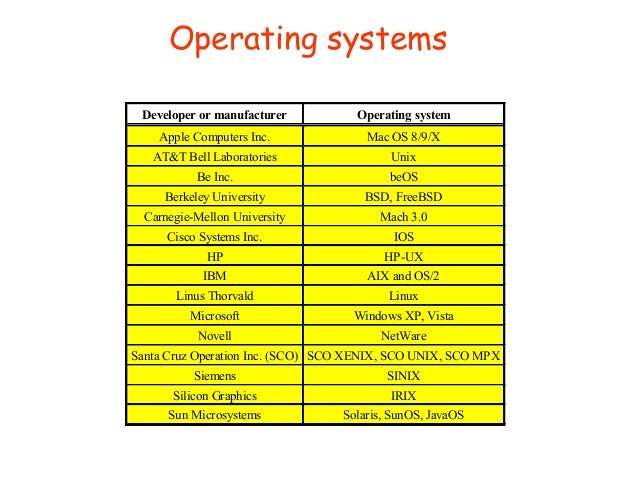
If additional samples of the same size were taken, you would begin to see how the possible estimates (possible values of the statistic) vary and how close they tend to be to the parameter value. However, a sample chosen at random will not necessarily yield an estimate (or statistic) that is exactly equal to the corresponding parameter for the population the next selected sample of the same size will probably give a different estimate from the first one. A statistic is used to estimate the corresponding value for a population (that is, a sample statistic estimates a population parameter). When a sample is selected from a population, a summary number can be computed from the observations resulting in the value of a statistic. Overview: Statistical inference is the process of drawing conclusions about a population parameter based on data. The distribution of the sample mean has a nice feature in which the sample mean will have a normal distribution if some conditions are met. However, all hope is not lost for doing this hypothesis test. When Jack creates a histogram of the salaries for a sample of players he notices that this distribution is not normal. This test requires that the distribution of salaries have a normal distribution. Application: Jack is a researcher who wants to perform a hypothesis test to learn something about the mean salary for all Major League Baseball players. The generated sample statistics can be examined to learn about properties of the sampling distribution of the statistic. In this lab, you will simulate random samples from a known population distribution and compute a sample statistic for each of the generated samples. Lab 6: Sampling Distributions and the CLT Objective: The objective of this lab is to give you a hands-‐on discussion and understanding of sampling distributions and the Central Limit Theorem (CLT), a theorem that plays an important role in statistics.
